
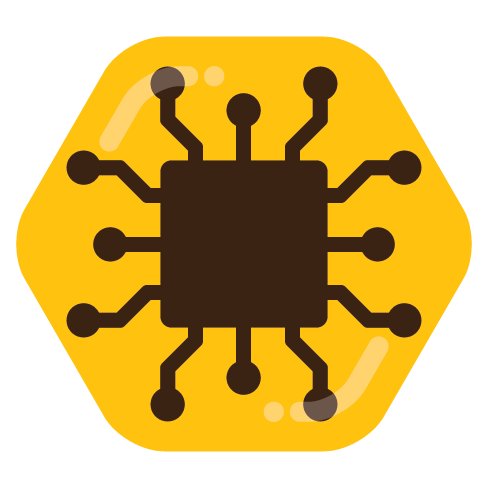
I have never, ever heard this definition of Luddite.
Formerly u/CanadaPlus101 on Reddit.
I have never, ever heard this definition of Luddite.
Yes, that wasn’t a random example for anyone OOTL. The thing the OG Luddites would do is break into factories and smash mechanical looms. They wanted to keep doing it the medieval way where you’re just crossing threads by hand over and over again, because “muh jerbs”.
I think that’s standard, actually. I can always do it at least.
Can confirm. My server has had federation issues, and when that has happened you can still post or vote on outside communities, but it’s not mirrored anywhere else.
Yes, sorry, I didn’t realise I was replying to the same user twice.
The problem was never people using AI to do the “heavy lifting” to increase their productivity by 50%; it was instead people increasing the output by 900%, and submitting ten really shitty pics or paragraphs, that look a lot like someone else’s, instead of a decent and original one.
Exactly. I guess I’m conditioned to expect “AI is smoke and mirrors” type comments, and that’s not true. They’re genuinely quite impressive and can make intuitive leaps they weren’t directly trained for. What they’re not is aligned; they just want to create human-like output, regardless of truth, greater context or morality, because that’s the only way we know how to train them.
I definitely hate searching something, and finding a website that almost reads as human with fake “authors”, but provides no useful information. And I really worry for people who are less experienced spotting AI errors and filler. That’s a moral issue, though, as opposed to a practical one; it seems to make ad money perfectly well for the “creators”.
Regarding code, from your other comment: note that some Linux and *BSD distributions banned AI submissions, like Gentoo and NetBSD. I believe it to be the same deal as news or art.
TIL. They’re going to have trouble identifying rulebreakers if contributors use the tool correctly the way we’ve discussed, though.
Shouldn’t we be glad they’re publishing both viewpoints? (On a real, actively debated issue, I don’t mean the “both sides” shit)
Occasionally. If you aren’t even proofreading it that’s dumb, but it can do a lot of heavy lifting in collaboration with a real worker.
For coders, there’s actually hard data on that. You’re worth about a coder and a half using CoPilot or similar.
And if you find a way to lie without getting caught, you aren’t part of the problem anyway.
I was about to disagree, but that’s actually really interesting. Could you expand on that?
Yes, it’s very hyped and being overused. Eventually the bullshit artists will move on to the next buzzword, though, and then there’s plenty of tasks it is very good at where it will continue to grow.
It will fail. Downvote me if you must, but AI generated erotica is just as here as machine-woven textiles.
To be clear, I wasn’t talking about an actual picture generating model. It was raw GPT trained on just text, asked to write instructions for a paint program to output a unicorn. That’s more convincing because it’s multiple steps away from the basic task it was trained on. Here, I found the paper, it starts with unicorns and then starts exploring other images, and eventually they delve into way more detail than I actually read. There’s a video talk that goes with it.
The trick with trying to “make” an AI do semantics, is that we don’t know what semantics is, exactly. I mean, that’s kind of what we started out with (remember the old pattern-matching chatbots?) but simpler approaches often worked better. Even the Transformer block itself is barely more complicated than a plain feed-forward network. I don’t think that’s so much because neural nets are more efficient (they really aren’t) but because we were looking for an answer to a question we didn’t have.
I think the challenge going forwards is freeing all that know-how from the black box we’ve put it in, somehow. Assuming we do want to mess with something so dangerous if handled carelessly.
Yeah, sorry, I don’t want to invert burden of proof - or at least, I don’t want to ask anything unreasonable of you.
Okay, let’s talk just about the performance we measure - it wasn’t clear to me that’s what you mean from what you wrote. Natural language is inherently imprecise, so no bitterness intended, but in particular that’s how I read the section outside of the spoiler tag.
By some measures, it can do quite a bit of novel logic. I recall it drawing a unicorn using text commends in one published test, for example, which correctly had a horn, body and four legs. That requires combining concepts in a way that almost certainly isn’t directly in the training data, so it’s fair to say it’s not a mere search engine. Then again, sometimes it just doesn’t do what it’s asked, for example when adding two numbers - it will give a plausible looking result, but that’s all.
So, we have a blackbox, and we’re trying to decide if it could become an existential threat. Do we agree a computer just as smart as us probably would be? If so, that reduces to whether the blackbox could be just as smart as us eventually. Up until now, there’s been great reasons to say no, even about blackbox software. I know clippy could never have done it, because there’s forms of reasoning classical algorithms just couldn’t do, despite great effort - it doesn’t matter if clippy is closed source, because it was a classical algorithm.
On the other hand, what neural nets can’t do is a total unknown. GPT-n won’t add numbers directly, but it is able to correctly preform the steps, which you can show by putting it in a chain-of-thought framework. It just “chooses” not to, because that’s not how it was trained. GPT-n can’t organise a faction that threatens human autonomy, but we don’t know if that’s because it doesn’t know the steps, or because of the lack of memory and cost function to make it do that.
It’s a blackbox, there’s no known limits on what it could do, and it’s certain to be improved on quickly at least in some way. For this reason, I think it might become an existential threat, in some future iteration.
Chinese room, called it. Just with a dog instead.
I have this debate so often, I’m going to try something a bit different. Why don’t we start by laying down how LLMs do work. If you had to explain as fully as you could the algorithm we’re talking about, how would you do it?
Yeah, the short-term outlook doesn’t look too dangerous right now. LLMs can do a lot of things we thought wouldn’t happen for a long time, but they still have major issues and are running out of easy scalability.
That being said, there’s a lot of different training schemes or integrations with classical algorithms that could be tried. ChatGPT knows a scary amount of stuff (inb4 Chinese room), it just doesn’t have any incentive to use it except to mimic human-generated text. I’m not saying it’s going to happen, but I think it’s premature to write off the possibility of an AI with complex planning capabilities in the next decade or so.
The human brain has an estimated 100 trillion neuronal connections - so probably a good order of magnitude estimation for the parameter count of an AGI model.
Yeah, but a lot of those do things unrelated to higher reasoning. A small monkey is smarter than a moose, despite the moose obviously having way more synapses.
I don’t think you can rely on this kind of argument so heavily. A brain isn’t a muscle.
The good old JNU licence.
I actually really like the AR glasses idea. That said, They need to be open source and de-spookified, and there needs to be some kind of regulation that they can’t store or transmit images without first displaying a recording indicator.
It’s probably not going to happen like that, though, so I’m not mad existing ones have such bad battery lives.
Oh, it’s definitely marketing nonsense. The question is if they managed to hide a sane, usable product underneath it, or if they’ve added some kind of anti-user nonsense to prevent you from repurposing the button.